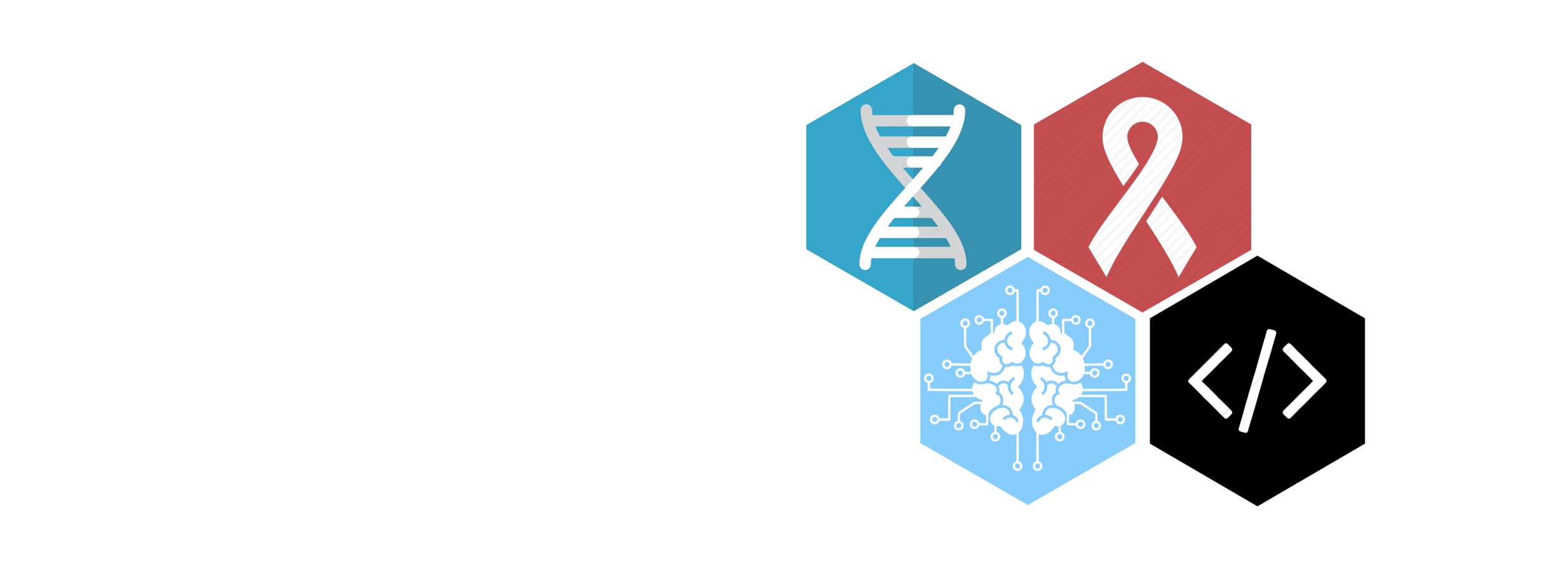
Research
We develop novel methods to extract insights from big data in genomics
Functional Genomics
Genome-wide association studies (GWAS) have identified tens of thousands of genetic variants associated with human diseases. Because the majority of GWAS variants fall into non-coding regions of the genome, their functional mechanism are usually not clear. Our group have used multi-omics method to study complex diseases. Using a combination of transcriptomic and epigenomic information, we have identified risk genes for coronary artery disease [Liu (2018) AJHG; Wirka (2019) Nature Medicine] and age-related macular degeneration [Liu (2019) Communications Biology]. Our group has a strong interest in expression quantitative trait loci (eQTL) mapping, and was part of the Genotype-Tissue Expression (GTEx) project [GTEx consortium (2017) Nature]. Our group is currently part of the Asian Immune Diversity Atlas (AIDA).
Cancer Genomics
Leptomeningeal disease (LMD) is a late-stage metastatic cancer in cerebrospinal fluid and the leptomeninges. The median survival time from LMD diagnosis is 3 - 5 months. Because patients with LMD do not undergo surgical resection, we sequenced circulating cells from patients’ cerebral spinal fluid. Upon comparison with solid brain tumor sample, we discovered a disproportionally low number of KRAS mutations and a high number of EGFR mutations. The median survival time for patients with EGFR mutations are shorter than those with the wild-type EGFR gene [Li (2018) Journal of Thoracic Oncology].
Data Science and Machine Learning
Novel scientific questions and data modalities require computational methods beyond existing ones. Our group develops statistical methods, machine learning models (especially deep learning models), and visualization techniques to fill these gaps. The computational techniques developed by our group are rooted in biological questions, but often borrow ideas from other domains such as natural language processing and computer vision. For the [Liu (2018) AJHG] paper, we developed a fast software to approximate sum of non-identical binomial random variables [Liu and Quertermous (2017), R Journal]. Combining microfluidic multiplex PCR and ancestry inference techniques, we developed the ANTseq pipeline to reduce the cost of ancestry determination by 5-fold [Liu (2016)].
Our group has a strong interest in deep learning. We developed a deep learning architecture to jointly model the cis- and trans-regulators of gene expression. Our method outperformed the previous state-of-the-art by as much as 20% [Liu (2017), NeurIPS]. Our group is also interested in the application of deep learning in the biomedical natural language processing. We developed ParaMed as the first biomedical English-Chinese machine translation dataset [Liu (2021) BMC Medical Informatics]. This dataset, combined with a state-of-the-art transformer architecture, outperformed baseline by 24 BLEU score (2-fold performance boost). We also showed that deep learning models underperform traditional rule-based methods in certain domains [Church and Liu (2021) Frontiers in Artificial Intelligence].